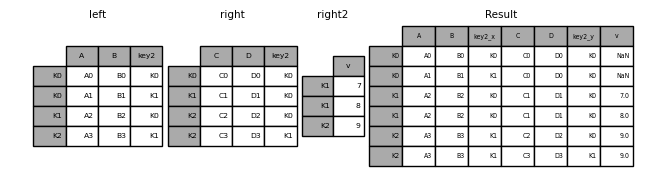
13 Data Reshaping and Enrichment
In this chapter, we will explore how to reshape and enrich data using pandas. Data reshaping and enriching are essential steps in data preprocessing because they help transform raw data into a more structured, useful, and meaningful format that can be effectively used for analysis or modeling.
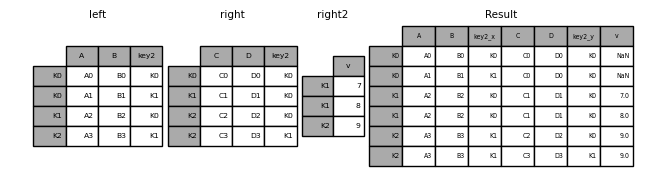
13.1 Data Reshaping
Data reshaping involves altering the structure of the data to suit the requirements of the analysis or modeling process. This is often needed when the data is in a format that is difficult to analyze or when it needs to be organized in a specific way.
13.1.1 Why Data Reshaping is Important:
Improves Data Organization: Raw data may be in a long format, where each observation is recorded on a separate row, or it may be in a wide format, where the same variable is spread across multiple columns. Reshaping allows you to reorganize the data into a format that is more suitable for analysis, such as turning multiple columns into a single column (melting) or aggregating rows into summary statistics (pivoting).
Facilitates Analysis: Some analysis techniques require specific data formats. For example, many machine learning algorithms require data in a matrix form, where each row represents a sample and each column represents a feature. Reshaping helps to convert data into this format.
Simplifies Visualization: Visualizing data is easier when it is in the right shape. For instance, plotting categorical data across time is easier when the data is in a tidy format, rather than having multiple variables spread across columns.
Efficient Grouping and Aggregation: In some cases, you may want to group data by certain categories or time periods, and reshaping can help aggregate data effectively for summarization.
13.1.2 Wide vs. Long Data Format in Data Reshaping
- Wide Format: Each variable in its own column; each row represents a unit, and data for multiple variables is spread across columns.
- Long Format: Variables are stacked into one column per variable, with additional columns used to indicate time, categories, or other distinctions, leading to a greater number of rows.
To reshape between these two formats, you can use pandas functions such as pivot_table()
, melt()
, stack()
, and unstack()
. Let’s next take a look at each of these methods one by one.
Throughout this section, we will continue using the gdp_lifeExpectancy.csv dataset. Let’s start by reading the CSV file into a pandas DataFrame.
import pandas as pd
import numpy as np
import seaborn as sns
import matplotlib.pyplot as plt
%matplotlib inline
# Load the data
= pd.read_csv('./Datasets/gdp_lifeExpectancy.csv')
gdp_lifeExp_data gdp_lifeExp_data.head()
country | continent | year | lifeExp | pop | gdpPercap | |
---|---|---|---|---|---|---|
0 | Afghanistan | Asia | 1952 | 28.801 | 8425333 | 779.445314 |
1 | Afghanistan | Asia | 1957 | 30.332 | 9240934 | 820.853030 |
2 | Afghanistan | Asia | 1962 | 31.997 | 10267083 | 853.100710 |
3 | Afghanistan | Asia | 1967 | 34.020 | 11537966 | 836.197138 |
4 | Afghanistan | Asia | 1972 | 36.088 | 13079460 | 739.981106 |
13.1.3 Pivoting “long” to “wide” using pivot_table()
In the last chapter, we learned that pivot_table()
is a powerful tool for performing groupwise aggregation in a structured format. The pivot_table()
function is used to reshape data by specifying columns for the index, columns, and values.
13.1.3.1 Pivoting a single column
Consider the life expectancy dataset. Let’s calculate the average life expectancy for each combination of country and year.
= gdp_lifeExp_data.pivot_table(index=['continent','country'], columns='year', values='lifeExp', aggfunc='mean')
gdp_lifeExp_data_pivot gdp_lifeExp_data_pivot.head()
year | 1952 | 1957 | 1962 | 1967 | 1972 | 1977 | 1982 | 1987 | 1992 | 1997 | 2002 | 2007 | |
---|---|---|---|---|---|---|---|---|---|---|---|---|---|
continent | country | ||||||||||||
Africa | Algeria | 43.077 | 45.685 | 48.303 | 51.407 | 54.518 | 58.014 | 61.368 | 65.799 | 67.744 | 69.152 | 70.994 | 72.301 |
Angola | 30.015 | 31.999 | 34.000 | 35.985 | 37.928 | 39.483 | 39.942 | 39.906 | 40.647 | 40.963 | 41.003 | 42.731 | |
Benin | 38.223 | 40.358 | 42.618 | 44.885 | 47.014 | 49.190 | 50.904 | 52.337 | 53.919 | 54.777 | 54.406 | 56.728 | |
Botswana | 47.622 | 49.618 | 51.520 | 53.298 | 56.024 | 59.319 | 61.484 | 63.622 | 62.745 | 52.556 | 46.634 | 50.728 | |
Burkina Faso | 31.975 | 34.906 | 37.814 | 40.697 | 43.591 | 46.137 | 48.122 | 49.557 | 50.260 | 50.324 | 50.650 | 52.295 |
Explanation
- values: Specifies the column to aggregate (e.g.,
lifeExp
in our example). - index: Groups by the rows based on the
continent
andcountry
column. - columns: Groups by the columns based on the
year
column. - aggfunc: Uses
mean
to calculate the average life expectancy.
With values of year
as columns, it is easy to compare any metric for different years.
#visualizing the change in life expectancy of all countries in 2007 as compared to that in 1957, i.e., the overall change in life expectancy in 50 years.
= gdp_lifeExp_data_pivot, x = 1957,y=2007,hue = 'continent')
sns.scatterplot(data = gdp_lifeExp_data_pivot, x = 1957,y = 1957); sns.lineplot(data
Observe that for some African countries, the life expectancy has decreased after 50 years. It is worth investigating these countries to identify factors associated with the decrease.
Another way to visualize a pivot table is by using a heatmap, which represents the 2D table as a matrix of colors, making it easier to interpret patterns and trends.
# Plotting a heatmap to visualize the life expectancy of all countries in all years, figure size is set to 15x10
=(10,10))
plt.figure(figsize"Life expectancy of all countries in all years")
plt.title(; sns.heatmap(gdp_lifeExp_data_pivot)
Common Aggregation Functions in pivot_table()
You can use various aggregation functions within pivot_table()
to summarize data:
mean
– Calculates the average of values within each group.sum
– Computes the total of values within each group.count
– Counts the number of non-null entries within each group.min
andmax
– Finds the minimum and maximum values within each group.
We can also define our own custom aggregation function and pass it to the aggfunc
parameter of the pivot_table()
function
For Example: Find the \(90^{th}\) percentile of life expectancy for each country and year combination
= gdp_lifeExp_data, values = 'lifeExp',index = 'country', columns ='year',aggfunc = lambda x:np.percentile(x,90)) pd.pivot_table(data
year | 1952 | 1957 | 1962 | 1967 | 1972 | 1977 | 1982 | 1987 | 1992 | 1997 | 2002 | 2007 |
---|---|---|---|---|---|---|---|---|---|---|---|---|
country | ||||||||||||
Afghanistan | 28.801 | 30.332 | 31.997 | 34.020 | 36.088 | 38.438 | 39.854 | 40.822 | 41.674 | 41.763 | 42.129 | 43.828 |
Albania | 55.230 | 59.280 | 64.820 | 66.220 | 67.690 | 68.930 | 70.420 | 72.000 | 71.581 | 72.950 | 75.651 | 76.423 |
Algeria | 43.077 | 45.685 | 48.303 | 51.407 | 54.518 | 58.014 | 61.368 | 65.799 | 67.744 | 69.152 | 70.994 | 72.301 |
Angola | 30.015 | 31.999 | 34.000 | 35.985 | 37.928 | 39.483 | 39.942 | 39.906 | 40.647 | 40.963 | 41.003 | 42.731 |
Argentina | 62.485 | 64.399 | 65.142 | 65.634 | 67.065 | 68.481 | 69.942 | 70.774 | 71.868 | 73.275 | 74.340 | 75.320 |
... | ... | ... | ... | ... | ... | ... | ... | ... | ... | ... | ... | ... |
Vietnam | 40.412 | 42.887 | 45.363 | 47.838 | 50.254 | 55.764 | 58.816 | 62.820 | 67.662 | 70.672 | 73.017 | 74.249 |
West Bank and Gaza | 43.160 | 45.671 | 48.127 | 51.631 | 56.532 | 60.765 | 64.406 | 67.046 | 69.718 | 71.096 | 72.370 | 73.422 |
Yemen, Rep. | 32.548 | 33.970 | 35.180 | 36.984 | 39.848 | 44.175 | 49.113 | 52.922 | 55.599 | 58.020 | 60.308 | 62.698 |
Zambia | 42.038 | 44.077 | 46.023 | 47.768 | 50.107 | 51.386 | 51.821 | 50.821 | 46.100 | 40.238 | 39.193 | 42.384 |
Zimbabwe | 48.451 | 50.469 | 52.358 | 53.995 | 55.635 | 57.674 | 60.363 | 62.351 | 60.377 | 46.809 | 39.989 | 43.487 |
142 rows × 12 columns
13.1.4 Melting “wide” to “long” using melt()
Melting is the inverse of pivoting. It transforms columns into rows. The melt()
function is used when you want to unpivot a DataFrame, turning multiple columns into rows.
Let’s first consider gdp_lifeExp_data_pivot created in the previous section
gdp_lifeExp_data_pivot.head()
year | 1952 | 1957 | 1962 | 1967 | 1972 | 1977 | 1982 | 1987 | 1992 | 1997 | 2002 | 2007 | |
---|---|---|---|---|---|---|---|---|---|---|---|---|---|
continent | country | ||||||||||||
Africa | Algeria | 43.077 | 45.685 | 48.303 | 51.407 | 54.518 | 58.014 | 61.368 | 65.799 | 67.744 | 69.152 | 70.994 | 72.301 |
Angola | 30.015 | 31.999 | 34.000 | 35.985 | 37.928 | 39.483 | 39.942 | 39.906 | 40.647 | 40.963 | 41.003 | 42.731 | |
Benin | 38.223 | 40.358 | 42.618 | 44.885 | 47.014 | 49.190 | 50.904 | 52.337 | 53.919 | 54.777 | 54.406 | 56.728 | |
Botswana | 47.622 | 49.618 | 51.520 | 53.298 | 56.024 | 59.319 | 61.484 | 63.622 | 62.745 | 52.556 | 46.634 | 50.728 | |
Burkina Faso | 31.975 | 34.906 | 37.814 | 40.697 | 43.591 | 46.137 | 48.122 | 49.557 | 50.260 | 50.324 | 50.650 | 52.295 |
='lifeExp', var_name='year', ignore_index=False) gdp_lifeExp_data_pivot.melt(value_name
year | lifeExp | ||
---|---|---|---|
continent | country | ||
Africa | Algeria | 1952 | 43.077 |
Angola | 1952 | 30.015 | |
Benin | 1952 | 38.223 | |
Botswana | 1952 | 47.622 | |
Burkina Faso | 1952 | 31.975 | |
... | ... | ... | ... |
Europe | Switzerland | 2007 | 81.701 |
Turkey | 2007 | 71.777 | |
United Kingdom | 2007 | 79.425 | |
Oceania | Australia | 2007 | 81.235 |
New Zealand | 2007 | 80.204 |
1704 rows × 2 columns
With the above DataFrame, we can visualize the mean life expectancy against year separately for each country in each continent.
13.1.5 Stacking and Unstacking using stack()
and unstack()
Stacking and unstacking are powerful techniques used to reshape DataFrames by moving data between rows and columns. These operations are particularly useful when working with multi-level index or multi-level columns, where the data is structured in a hierarchical format.
stack()
: Converts columns into rows, which means it “stacks” the data into a long format.unstack()
: Converts rows into columns, reversing the effect ofstack()
.
Let’s use the GDP per capita dataset and create a DataFrame with a multi-level index (e.g., continent, country) and multi-level columns (e.g., lifeExp , gdpPercap, and pop).
# calculate the average and standard deviation of life expectancy, population, and GDP per capita for countries across the years.
= gdp_lifeExp_data.groupby(['continent', 'country'])[['lifeExp', 'pop', 'gdpPercap']].agg(['mean', 'std'])
gdp_lifeExp_multilevel_data gdp_lifeExp_multilevel_data
lifeExp | pop | gdpPercap | |||||
---|---|---|---|---|---|---|---|
mean | std | mean | std | mean | std | ||
continent | country | ||||||
Africa | Algeria | 59.030167 | 10.340069 | 1.987541e+07 | 8.613355e+06 | 4426.025973 | 1310.337656 |
Angola | 37.883500 | 4.005276 | 7.309390e+06 | 2.672281e+06 | 3607.100529 | 1165.900251 | |
Benin | 48.779917 | 6.128681 | 4.017497e+06 | 2.105002e+06 | 1155.395107 | 159.741306 | |
Botswana | 54.597500 | 5.929476 | 9.711862e+05 | 4.710965e+05 | 5031.503557 | 4178.136987 | |
Burkina Faso | 44.694000 | 6.845792 | 7.548677e+06 | 3.247808e+06 | 843.990665 | 183.430087 | |
... | ... | ... | ... | ... | ... | ... | ... |
Europe | Switzerland | 75.565083 | 4.011572 | 6.384293e+06 | 8.582009e+05 | 27074.334405 | 6886.463308 |
Turkey | 59.696417 | 9.030591 | 4.590901e+07 | 1.667768e+07 | 4469.453380 | 2049.665102 | |
United Kingdom | 73.922583 | 3.378943 | 5.608780e+07 | 3.174339e+06 | 19380.472986 | 7388.189399 | |
Oceania | Australia | 74.662917 | 4.147774 | 1.464931e+07 | 3.915203e+06 | 19980.595634 | 7815.405220 |
New Zealand | 73.989500 | 3.559724 | 3.100032e+06 | 6.547108e+05 | 17262.622813 | 4409.009167 |
142 rows × 6 columns
The resulting DataFrame has two levels of index and two levels of columns, as shown below:
# check the level of row and column index
gdp_lifeExp_multilevel_data.index.nlevels
2
gdp_lifeExp_multilevel_data.columns.nlevels
2
if you want to see the data along with the index and columns (in a more accessible way), you can directly print the .index
and .columns
attributes.
gdp_lifeExp_multilevel_data.index
MultiIndex([( 'Africa', 'Algeria'),
( 'Africa', 'Angola'),
( 'Africa', 'Benin'),
( 'Africa', 'Botswana'),
( 'Africa', 'Burkina Faso'),
( 'Africa', 'Burundi'),
( 'Africa', 'Cameroon'),
( 'Africa', 'Central African Republic'),
( 'Africa', 'Chad'),
( 'Africa', 'Comoros'),
...
( 'Europe', 'Serbia'),
( 'Europe', 'Slovak Republic'),
( 'Europe', 'Slovenia'),
( 'Europe', 'Spain'),
( 'Europe', 'Sweden'),
( 'Europe', 'Switzerland'),
( 'Europe', 'Turkey'),
( 'Europe', 'United Kingdom'),
('Oceania', 'Australia'),
('Oceania', 'New Zealand')],
names=['continent', 'country'], length=142)
gdp_lifeExp_multilevel_data.columns
MultiIndex([( 'lifeExp', 'mean'),
( 'lifeExp', 'std'),
( 'pop', 'mean'),
( 'pop', 'std'),
('gdpPercap', 'mean'),
('gdpPercap', 'std')],
)
As seen, the index and columns each contain tuples. This is the reason that when performing data subsetting on a DataFrame with multi-level index and columns, you need to supply a tuple to specify the exact location of the data.
'Americas', 'United States'), ('lifeExp', 'mean')] gdp_lifeExp_multilevel_data.loc[(
73.4785
gdp_lifeExp_multilevel_data.columns.values
array([('lifeExp', 'mean'), ('lifeExp', 'std'), ('pop', 'mean'),
('pop', 'std'), ('gdpPercap', 'mean'), ('gdpPercap', 'std')],
dtype=object)
13.1.5.1 Understanding the level
in multi-level index and columns
The gdp_lifeExp_multilevel_data
dataframe have two levels of index and two level of columns, You can reference these levels in various operations, such as grouping, subsetting, or performing aggregations. The level
parameter helps identify which part of the multi-level structure you’re working with.
0) gdp_lifeExp_multilevel_data.index.get_level_values(
Index(['Africa', 'Africa', 'Africa', 'Africa', 'Africa', 'Africa', 'Africa',
'Africa', 'Africa', 'Africa',
...
'Europe', 'Europe', 'Europe', 'Europe', 'Europe', 'Europe', 'Europe',
'Europe', 'Oceania', 'Oceania'],
dtype='object', name='continent', length=142)
1) gdp_lifeExp_multilevel_data.index.get_level_values(
0) gdp_lifeExp_multilevel_data.columns.get_level_values(
Index(['lifeExp', 'lifeExp', 'pop', 'pop', 'gdpPercap', 'gdpPercap'], dtype='object')
1) gdp_lifeExp_multilevel_data.columns.get_level_values(
Index(['mean', 'std', 'mean', 'std', 'mean', 'std'], dtype='object')
-1) gdp_lifeExp_multilevel_data.columns.get_level_values(
Index(['mean', 'std', 'mean', 'std', 'mean', 'std'], dtype='object')
As seen above, the outermost level corresponds to level=0
, and it increases as we move inward to the inner levels. The innermost level can be referred to as -1
.
Now, let’s use the level number in the droplevel()
method to see how it works. The syntax for this method is as follows:
DataFrame.droplevel(level, axis=0)
# drop the first level of row index
0, axis=0) gdp_lifeExp_multilevel_data.droplevel(
lifeExp | pop | gdpPercap | ||||
---|---|---|---|---|---|---|
mean | std | mean | std | mean | std | |
country | ||||||
Algeria | 59.030167 | 10.340069 | 1.987541e+07 | 8.613355e+06 | 4426.025973 | 1310.337656 |
Angola | 37.883500 | 4.005276 | 7.309390e+06 | 2.672281e+06 | 3607.100529 | 1165.900251 |
Benin | 48.779917 | 6.128681 | 4.017497e+06 | 2.105002e+06 | 1155.395107 | 159.741306 |
Botswana | 54.597500 | 5.929476 | 9.711862e+05 | 4.710965e+05 | 5031.503557 | 4178.136987 |
Burkina Faso | 44.694000 | 6.845792 | 7.548677e+06 | 3.247808e+06 | 843.990665 | 183.430087 |
... | ... | ... | ... | ... | ... | ... |
Switzerland | 75.565083 | 4.011572 | 6.384293e+06 | 8.582009e+05 | 27074.334405 | 6886.463308 |
Turkey | 59.696417 | 9.030591 | 4.590901e+07 | 1.667768e+07 | 4469.453380 | 2049.665102 |
United Kingdom | 73.922583 | 3.378943 | 5.608780e+07 | 3.174339e+06 | 19380.472986 | 7388.189399 |
Australia | 74.662917 | 4.147774 | 1.464931e+07 | 3.915203e+06 | 19980.595634 | 7815.405220 |
New Zealand | 73.989500 | 3.559724 | 3.100032e+06 | 6.547108e+05 | 17262.622813 | 4409.009167 |
142 rows × 6 columns
# drop the first level of column index
0, axis=1) gdp_lifeExp_multilevel_data.droplevel(
mean | std | mean | std | mean | std | ||
---|---|---|---|---|---|---|---|
continent | country | ||||||
Africa | Algeria | 59.030167 | 10.340069 | 1.987541e+07 | 8.613355e+06 | 4426.025973 | 1310.337656 |
Angola | 37.883500 | 4.005276 | 7.309390e+06 | 2.672281e+06 | 3607.100529 | 1165.900251 | |
Benin | 48.779917 | 6.128681 | 4.017497e+06 | 2.105002e+06 | 1155.395107 | 159.741306 | |
Botswana | 54.597500 | 5.929476 | 9.711862e+05 | 4.710965e+05 | 5031.503557 | 4178.136987 | |
Burkina Faso | 44.694000 | 6.845792 | 7.548677e+06 | 3.247808e+06 | 843.990665 | 183.430087 | |
... | ... | ... | ... | ... | ... | ... | ... |
Europe | Switzerland | 75.565083 | 4.011572 | 6.384293e+06 | 8.582009e+05 | 27074.334405 | 6886.463308 |
Turkey | 59.696417 | 9.030591 | 4.590901e+07 | 1.667768e+07 | 4469.453380 | 2049.665102 | |
United Kingdom | 73.922583 | 3.378943 | 5.608780e+07 | 3.174339e+06 | 19380.472986 | 7388.189399 | |
Oceania | Australia | 74.662917 | 4.147774 | 1.464931e+07 | 3.915203e+06 | 19980.595634 | 7815.405220 |
New Zealand | 73.989500 | 3.559724 | 3.100032e+06 | 6.547108e+05 | 17262.622813 | 4409.009167 |
142 rows × 6 columns
13.1.5.2 Stacking (Columns to Rows ): stack()
The stack()
function pivots the columns into rows. You can specify which level you want to stack using the level
parameter. By default, it will stack the innermost level of the columns.
=True) gdp_lifeExp_multilevel_data.stack(future_stack
lifeExp | pop | gdpPercap | |||
---|---|---|---|---|---|
continent | country | ||||
Africa | Algeria | mean | 59.030167 | 1.987541e+07 | 4426.025973 |
std | 10.340069 | 8.613355e+06 | 1310.337656 | ||
Angola | mean | 37.883500 | 7.309390e+06 | 3607.100529 | |
std | 4.005276 | 2.672281e+06 | 1165.900251 | ||
Benin | mean | 48.779917 | 4.017497e+06 | 1155.395107 | |
... | ... | ... | ... | ... | ... |
Europe | United Kingdom | std | 3.378943 | 3.174339e+06 | 7388.189399 |
Oceania | Australia | mean | 74.662917 | 1.464931e+07 | 19980.595634 |
std | 4.147774 | 3.915203e+06 | 7815.405220 | ||
New Zealand | mean | 73.989500 | 3.100032e+06 | 17262.622813 | |
std | 3.559724 | 6.547108e+05 | 4409.009167 |
284 rows × 3 columns
Let’s change the default setting by specifying the level=0
(the outmost level)
=0, future_stack=True) gdp_lifeExp_multilevel_data.stack(level
mean | std | |||
---|---|---|---|---|
continent | country | |||
Africa | Algeria | lifeExp | 5.903017e+01 | 1.034007e+01 |
pop | 1.987541e+07 | 8.613355e+06 | ||
gdpPercap | 4.426026e+03 | 1.310338e+03 | ||
Angola | lifeExp | 3.788350e+01 | 4.005276e+00 | |
pop | 7.309390e+06 | 2.672281e+06 | ||
... | ... | ... | ... | ... |
Oceania | Australia | pop | 1.464931e+07 | 3.915203e+06 |
gdpPercap | 1.998060e+04 | 7.815405e+03 | ||
New Zealand | lifeExp | 7.398950e+01 | 3.559724e+00 | |
pop | 3.100032e+06 | 6.547108e+05 | ||
gdpPercap | 1.726262e+04 | 4.409009e+03 |
426 rows × 2 columns
13.1.5.3 Unstacking (Rows to Columns) : unstack()
The unstack()
function pivots the rows back into columns. By default, it will unstack the innermost level of the index.
Let’s reverse the prevous dataframe to its original shape using unstack()
.
=0, future_stack=True).unstack() gdp_lifeExp_multilevel_data.stack(level
mean | std | ||||||
---|---|---|---|---|---|---|---|
lifeExp | pop | gdpPercap | lifeExp | pop | gdpPercap | ||
continent | country | ||||||
Africa | Algeria | 59.030167 | 1.987541e+07 | 4426.025973 | 10.340069 | 8.613355e+06 | 1310.337656 |
Angola | 37.883500 | 7.309390e+06 | 3607.100529 | 4.005276 | 2.672281e+06 | 1165.900251 | |
Benin | 48.779917 | 4.017497e+06 | 1155.395107 | 6.128681 | 2.105002e+06 | 159.741306 | |
Botswana | 54.597500 | 9.711862e+05 | 5031.503557 | 5.929476 | 4.710965e+05 | 4178.136987 | |
Burkina Faso | 44.694000 | 7.548677e+06 | 843.990665 | 6.845792 | 3.247808e+06 | 183.430087 | |
... | ... | ... | ... | ... | ... | ... | ... |
Europe | Switzerland | 75.565083 | 6.384293e+06 | 27074.334405 | 4.011572 | 8.582009e+05 | 6886.463308 |
Turkey | 59.696417 | 4.590901e+07 | 4469.453380 | 9.030591 | 1.667768e+07 | 2049.665102 | |
United Kingdom | 73.922583 | 5.608780e+07 | 19380.472986 | 3.378943 | 3.174339e+06 | 7388.189399 | |
Oceania | Australia | 74.662917 | 1.464931e+07 | 19980.595634 | 4.147774 | 3.915203e+06 | 7815.405220 |
New Zealand | 73.989500 | 3.100032e+06 | 17262.622813 | 3.559724 | 6.547108e+05 | 4409.009167 |
142 rows × 6 columns
13.1.6 Transposing using .T
attribute
If you simply want to swap rows and columns, you can use the .T
attribute.
gdp_lifeExp_multilevel_data.T
continent | Africa | ... | Europe | Oceania | ||||||||||||||||||
---|---|---|---|---|---|---|---|---|---|---|---|---|---|---|---|---|---|---|---|---|---|---|
country | Algeria | Angola | Benin | Botswana | Burkina Faso | Burundi | Cameroon | Central African Republic | Chad | Comoros | ... | Serbia | Slovak Republic | Slovenia | Spain | Sweden | Switzerland | Turkey | United Kingdom | Australia | New Zealand | |
lifeExp | mean | 5.903017e+01 | 3.788350e+01 | 4.877992e+01 | 54.597500 | 4.469400e+01 | 4.481733e+01 | 4.812850e+01 | 4.386692e+01 | 4.677358e+01 | 52.381750 | ... | 6.855100e+01 | 7.069608e+01 | 7.160075e+01 | 7.420342e+01 | 7.617700e+01 | 7.556508e+01 | 5.969642e+01 | 7.392258e+01 | 7.466292e+01 | 7.398950e+01 |
std | 1.034007e+01 | 4.005276e+00 | 6.128681e+00 | 5.929476 | 6.845792e+00 | 3.174882e+00 | 5.467960e+00 | 4.720690e+00 | 4.887978e+00 | 8.132353 | ... | 4.906736e+00 | 2.715787e+00 | 3.677979e+00 | 5.155859e+00 | 3.003990e+00 | 4.011572e+00 | 9.030591e+00 | 3.378943e+00 | 4.147774e+00 | 3.559724e+00 | |
pop | mean | 1.987541e+07 | 7.309390e+06 | 4.017497e+06 | 971186.166667 | 7.548677e+06 | 4.651608e+06 | 9.816648e+06 | 2.560963e+06 | 5.329256e+06 | 361683.916667 | ... | 8.783887e+06 | 4.774507e+06 | 1.794381e+06 | 3.585180e+07 | 8.220029e+06 | 6.384293e+06 | 4.590901e+07 | 5.608780e+07 | 1.464931e+07 | 3.100032e+06 |
std | 8.613355e+06 | 2.672281e+06 | 2.105002e+06 | 471096.527435 | 3.247808e+06 | 1.874538e+06 | 4.363640e+06 | 1.072158e+06 | 2.464304e+06 | 182998.737990 | ... | 1.192153e+06 | 6.425096e+05 | 2.022077e+05 | 4.323928e+06 | 6.365660e+05 | 8.582009e+05 | 1.667768e+07 | 3.174339e+06 | 3.915203e+06 | 6.547108e+05 | |
gdpPercap | mean | 4.426026e+03 | 3.607101e+03 | 1.155395e+03 | 5031.503557 | 8.439907e+02 | 4.716630e+02 | 1.774634e+03 | 9.587847e+02 | 1.165454e+03 | 1314.380339 | ... | 9.305049e+03 | 1.041553e+04 | 1.407458e+04 | 1.402983e+04 | 1.994313e+04 | 2.707433e+04 | 4.469453e+03 | 1.938047e+04 | 1.998060e+04 | 1.726262e+04 |
std | 1.310338e+03 | 1.165900e+03 | 1.597413e+02 | 4178.136987 | 1.834301e+02 | 9.932972e+01 | 4.195899e+02 | 1.919529e+02 | 2.305481e+02 | 298.334389 | ... | 3.829907e+03 | 3.650231e+03 | 6.470288e+03 | 8.046635e+03 | 7.723248e+03 | 6.886463e+03 | 2.049665e+03 | 7.388189e+03 | 7.815405e+03 | 4.409009e+03 |
6 rows × 142 columns
13.1.7 Summary of Common Reshaping Functions
Function | Description | Example |
---|---|---|
pivot() |
Reshape data by creating new columns from existing ones. | Pivot data on index/columns. |
melt() |
Unpivot data, turning columns into rows. | Convert wide format to long. |
stack() |
Convert columns to rows. | Move column data to rows. |
unstack() |
Convert rows back to columns. | Move row data to columns. |
.T |
Transpose the DataFrame (swap rows and columns). | Transpose the entire DataFrame. |
Practical Use Cases:
- Pivoting: Useful for time series analysis or when you want to group and summarize data by specific categories.
- Melting: Helpful when you need to reshape wide data for easier analysis or when preparing data for machine learning algorithms.
- Stacking/Unstacking: Useful when you are working with hierarchical index DataFrames.
- Transpose: Helpful for reorienting data for analysis or visualization.
13.1.8 Converting from Multi-Level Index to Single-Level Index DataFrame
If you’re uncomfortable working with DataFrames that have multi-level indexes and columns, you can convert them into single-level DataFrames using the methods you learned in the previous chapter:
reset_index()
- Flattening the multi-level columns
# reset the index to convert the multi-level index to a single-level index
gdp_lifeExp_multilevel_data.reset_index()
continent | country | lifeExp | pop | gdpPercap | ||||
---|---|---|---|---|---|---|---|---|
mean | std | mean | std | mean | std | |||
0 | Africa | Algeria | 59.030167 | 10.340069 | 1.987541e+07 | 8.613355e+06 | 4426.025973 | 1310.337656 |
1 | Africa | Angola | 37.883500 | 4.005276 | 7.309390e+06 | 2.672281e+06 | 3607.100529 | 1165.900251 |
2 | Africa | Benin | 48.779917 | 6.128681 | 4.017497e+06 | 2.105002e+06 | 1155.395107 | 159.741306 |
3 | Africa | Botswana | 54.597500 | 5.929476 | 9.711862e+05 | 4.710965e+05 | 5031.503557 | 4178.136987 |
4 | Africa | Burkina Faso | 44.694000 | 6.845792 | 7.548677e+06 | 3.247808e+06 | 843.990665 | 183.430087 |
... | ... | ... | ... | ... | ... | ... | ... | ... |
137 | Europe | Switzerland | 75.565083 | 4.011572 | 6.384293e+06 | 8.582009e+05 | 27074.334405 | 6886.463308 |
138 | Europe | Turkey | 59.696417 | 9.030591 | 4.590901e+07 | 1.667768e+07 | 4469.453380 | 2049.665102 |
139 | Europe | United Kingdom | 73.922583 | 3.378943 | 5.608780e+07 | 3.174339e+06 | 19380.472986 | 7388.189399 |
140 | Oceania | Australia | 74.662917 | 4.147774 | 1.464931e+07 | 3.915203e+06 | 19980.595634 | 7815.405220 |
141 | Oceania | New Zealand | 73.989500 | 3.559724 | 3.100032e+06 | 6.547108e+05 | 17262.622813 | 4409.009167 |
142 rows × 8 columns
# flatten the multi-level column
= ['_'.join(col).strip() for col in gdp_lifeExp_multilevel_data.columns.values]
gdp_lifeExp_multilevel_data.columns gdp_lifeExp_multilevel_data
lifeExp_mean | lifeExp_std | pop_mean | pop_std | gdpPercap_mean | gdpPercap_std | ||
---|---|---|---|---|---|---|---|
continent | country | ||||||
Africa | Algeria | 59.030167 | 10.340069 | 1.987541e+07 | 8.613355e+06 | 4426.025973 | 1310.337656 |
Angola | 37.883500 | 4.005276 | 7.309390e+06 | 2.672281e+06 | 3607.100529 | 1165.900251 | |
Benin | 48.779917 | 6.128681 | 4.017497e+06 | 2.105002e+06 | 1155.395107 | 159.741306 | |
Botswana | 54.597500 | 5.929476 | 9.711862e+05 | 4.710965e+05 | 5031.503557 | 4178.136987 | |
Burkina Faso | 44.694000 | 6.845792 | 7.548677e+06 | 3.247808e+06 | 843.990665 | 183.430087 | |
... | ... | ... | ... | ... | ... | ... | ... |
Europe | Switzerland | 75.565083 | 4.011572 | 6.384293e+06 | 8.582009e+05 | 27074.334405 | 6886.463308 |
Turkey | 59.696417 | 9.030591 | 4.590901e+07 | 1.667768e+07 | 4469.453380 | 2049.665102 | |
United Kingdom | 73.922583 | 3.378943 | 5.608780e+07 | 3.174339e+06 | 19380.472986 | 7388.189399 | |
Oceania | Australia | 74.662917 | 4.147774 | 1.464931e+07 | 3.915203e+06 | 19980.595634 | 7815.405220 |
New Zealand | 73.989500 | 3.559724 | 3.100032e+06 | 6.547108e+05 | 17262.622813 | 4409.009167 |
142 rows × 6 columns
13.2 Data Enriching
Data enriching involves enhancing your existing dataset by adding additional data, often from external sources, to make your dataset more insightful.
There are 3 common methods for data enriching:
- Merging using
merge()
: Combining datasets based on a common key. - Joining using
join()
: Using indices to combine data. - Concatenation using
concat()
: Stacking DataFrames vertically or horizontally.
Let’s first load the existing data
= pd.read_csv('./datasets/LOTR.csv')
df_existing print(df_existing.shape)
df_existing.head()
(4, 3)
FellowshipID | FirstName | Skills | |
---|---|---|---|
0 | 1001 | Frodo | Hiding |
1 | 1002 | Samwise | Gardening |
2 | 1003 | Gandalf | Spells |
3 | 1004 | Pippin | Fireworks |
Let’s next load the external data we want to combine to the existing data
= pd.read_csv("./datasets/LOTR 2.csv")
df_external print(df_external.shape)
df_external.head()
(5, 3)
FellowshipID | FirstName | Age | |
---|---|---|---|
0 | 1001 | Frodo | 50 |
1 | 1002 | Samwise | 39 |
2 | 1006 | Legolas | 2931 |
3 | 1007 | Elrond | 6520 |
4 | 1008 | Barromir | 51 |
13.2.1 Combining based on common keys: merge()
= pd.merge(df_existing, df_external)
df_enriched_merge print(df_enriched_merge.shape)
df_enriched_merge.head()
(2, 4)
FellowshipID | FirstName | Skills | Age | |
---|---|---|---|---|
0 | 1001 | Frodo | Hiding | 50 |
1 | 1002 | Samwise | Gardening | 39 |
You can also do this way
= df_existing.merge(df_external)
df_enriched_merge print(df_enriched_merge.shape)
df_enriched_merge.head()
(2, 4)
FellowshipID | FirstName | Skills | Age | |
---|---|---|---|---|
0 | 1001 | Frodo | Hiding | 50 |
1 | 1002 | Samwise | Gardening | 39 |
By default, the merge()
function in pandas performs an inner join, which combines two DataFrames based on the common keys shared by both. Only the rows with matching keys in both DataFrames are retained in the result.
This behavior can be observed in the following code:
= pd.merge(df_existing, df_external, how='inner')
df_enriched_merge print(df_enriched_merge.shape)
df_enriched_merge.head()
(2, 4)
FellowshipID | FirstName | Skills | Age | |
---|---|---|---|---|
0 | 1001 | Frodo | Hiding | 50 |
1 | 1002 | Samwise | Gardening | 39 |
When you may use inner join? You should use inner join when you cannot carry out the analysis unless the observation corresponding to the key column(s) is present in both the tables.
Example: Suppose you wish to analyze the association between vaccinations and covid infection rate based on country-level data. In one of the datasets, you have the infection rate for each country, while in the other one you have the number of vaccinations in each country. The countries which have either the vaccination or the infection rate missing, cannot help analyze the association. In such as case you may be interested only in countries that have values for both the variables. Thus, you will use inner join to discard the countries with either value missing.
In merge()
, the how
parameter specifies the type of merge to be performed, the default option is inner
, it could also be left
, right
, and outer
.
These join types give you flexibility in how you want to merge and combine data from different sources.
Let’s see how each of these works using our dataframes defined above
Left Join
- Returns all rows from the left DataFrame and the matching rows from the right DataFrame.
- Missing values (
NaN
) are introduced for non-matching keys from the right DataFrame.
= pd.merge(df_existing, df_external, how='left')
df_merge_lft print(df_merge_lft.shape)
df_merge_lft.head()
(4, 4)
FellowshipID | FirstName | Skills | Age | |
---|---|---|---|---|
0 | 1001 | Frodo | Hiding | 50.0 |
1 | 1002 | Samwise | Gardening | 39.0 |
2 | 1003 | Gandalf | Spells | NaN |
3 | 1004 | Pippin | Fireworks | NaN |
When you may use left join? You should use left join when the primary variable(s) of interest are present in the one of the datasets, and whose missing values cannot be imputed. The variable(s) in the other dataset may not be as important or it may be possible to reasonably impute their values, if missing corresponding to the observation in the primary dataset.
Examples:
Suppose you wish to analyze the association between the covid infection rate and the government effectiveness score (a metric used to determine the effectiveness of the government in implementing policies, upholding law and order etc.) based on the data of all countries. Let us say that one of the datasets contains the covid infection rate, while the other one contains the government effectiveness score for each country. If the infection rate for a country is missing, it might be hard to impute. However, the government effectiveness score may be easier to impute based on GDP per capita, crime rate etc. - information that is easily available online. In such a case, you may wish to use a left join where you keep all the countries for which the infection rate is known.
Suppose you wish to analyze the association between demographics such as age, income etc. and the amount of credit card spend. Let us say one of the datasets contains the demographic information of each customer, while the other one contains the credit card spend for the customers who made at least one purchase. In such as case, you may want to do a left join as customers not making any purchase might be absent in the card spend data. Their spend can be imputed as zero after merging the datasets.
Right join
- Returns all rows from the right DataFrame and the matching rows from the left DataFrame.
- Missing values (
NaN
) are introduced for non-matching keys from the left DataFrame.
= pd.merge(df_existing, df_external, how='right')
df_merge_right print(df_merge_right.shape)
df_merge_right.head()
(5, 4)
FellowshipID | FirstName | Skills | Age | |
---|---|---|---|---|
0 | 1001 | Frodo | Hiding | 50 |
1 | 1002 | Samwise | Gardening | 39 |
2 | 1006 | Legolas | NaN | 2931 |
3 | 1007 | Elrond | NaN | 6520 |
4 | 1008 | Barromir | NaN | 51 |
When you may use right join? You can always use a left join instead of a right join. Their purpose is the same.
13.2.1.1 outer join
- Returns all rows from both DataFrames, with
NaN
values where no match is found.
= pd.merge(df_existing, df_external, how='outer')
df_merge_outer print(df_merge_outer.shape)
df_merge_outer
(7, 4)
FellowshipID | FirstName | Skills | Age | |
---|---|---|---|---|
0 | 1001 | Frodo | Hiding | 50.0 |
1 | 1002 | Samwise | Gardening | 39.0 |
2 | 1003 | Gandalf | Spells | NaN |
3 | 1004 | Pippin | Fireworks | NaN |
4 | 1006 | Legolas | NaN | 2931.0 |
5 | 1007 | Elrond | NaN | 6520.0 |
6 | 1008 | Barromir | NaN | 51.0 |
When you may use outer join? You should use an outer join when you cannot afford to lose data present in either of the tables. All the other joins may result in loss of data.
Example: Suppose I took two course surveys for this course. If I need to analyze student sentiment during the course, I will take an outer join of both the surveys. Assume that each survey is a dataset, where each row corresponds to a unique student. Even if a student has answered one of the two surverys, it will be indicative of the sentiment, and will be useful to keep in the merged dataset.
13.2.2 Combining based on Indices: join()
Unlike merge()
, join()
in pandas combines data based on the index. When using join()
, you must specify the suffixes
parameter if there are overlapping column names, as it does not have default values. You can refer to the official function definition here: pandas.DataFrame.join.
= df_existing.join(df_external)
df_enriched_join print(df_enriched_join.shape)
df_enriched_join.head()
ValueError: columns overlap but no suffix specified: Index(['FellowshipID', 'FirstName'], dtype='object')
Let’s set the suffix to fix this issue
= df_existing.join(df_external, lsuffix = '_existing',rsuffix = '_external')
df_enriched_join print(df_enriched_join.shape)
df_enriched_join.head()
(4, 6)
FellowshipID_existing | FirstName_existing | Skills | FellowshipID_external | FirstName_external | Age | |
---|---|---|---|---|---|---|
0 | 1001 | Frodo | Hiding | 1001 | Frodo | 50 |
1 | 1002 | Samwise | Gardening | 1002 | Samwise | 39 |
2 | 1003 | Gandalf | Spells | 1006 | Legolas | 2931 |
3 | 1004 | Pippin | Fireworks | 1007 | Elrond | 6520 |
As can be observed, join()
merges DataFrames using their index and performs a left join.
Now, let’s set the “FellowshipID” column as the index in both DataFrames and see what happens.
# set the index of the dataframes as the "FellowshipID" column
'FellowshipID', inplace=True)
df_existing.set_index('FellowshipID', inplace=True) df_external.set_index(
# join the two dataframes
= df_existing.join(df_external, lsuffix = '_existing',rsuffix = '_external')
df_enriched_join print(df_enriched_join.shape)
df_enriched_join
(4, 4)
FirstName_existing | Skills | FirstName_external | Age | |
---|---|---|---|---|
FellowshipID | ||||
1001 | Frodo | Hiding | Frodo | 50.0 |
1002 | Samwise | Gardening | Samwise | 39.0 |
1003 | Gandalf | Spells | NaN | NaN |
1004 | Pippin | Fireworks | NaN | NaN |
In this case, df_enriched_join
will contain all rows from df_existing
and the corresponding matching rows from df_external
, with NaN
values for non-matching entries.
Similar to merge()
, you can change the type of join in join()
by explicitly specifying the how
parameter. However, this explanation will be skipped here, as the different join types have already been covered in the merge()
section above.
13.2.3 Stacking vertically or horizontally: concat()
concat()
is used in pandas to concatenate DataFrames along a specified axis, either rows or columns. Similar to concat()
in NumPy, it defaults to concatenating along the rows.
# let's concatenate the two dataframes using default setting
= pd.concat([df_existing, df_external])
df_concat print(df_concat.shape)
df_concat
(9, 3)
FirstName | Skills | Age | |
---|---|---|---|
FellowshipID | |||
1001 | Frodo | Hiding | NaN |
1002 | Samwise | Gardening | NaN |
1003 | Gandalf | Spells | NaN |
1004 | Pippin | Fireworks | NaN |
1001 | Frodo | NaN | 50.0 |
1002 | Samwise | NaN | 39.0 |
1006 | Legolas | NaN | 2931.0 |
1007 | Elrond | NaN | 6520.0 |
1008 | Barromir | NaN | 51.0 |
# Concatenating along columns (horizontal)
= pd.concat([df_existing, df_external], axis=1)
df_concat_columns print(df_concat_columns.shape)
df_concat_columns
(7, 4)
FirstName | Skills | FirstName | Age | |
---|---|---|---|---|
FellowshipID | ||||
1001 | Frodo | Hiding | Frodo | 50.0 |
1002 | Samwise | Gardening | Samwise | 39.0 |
1003 | Gandalf | Spells | NaN | NaN |
1004 | Pippin | Fireworks | NaN | NaN |
1006 | NaN | NaN | Legolas | 2931.0 |
1007 | NaN | NaN | Elrond | 6520.0 |
1008 | NaN | NaN | Barromir | 51.0 |
13.2.4 Missing values after data enriching
Using either of the approaches mentioned above may introduce missing values for unmatched entries. Handling these missing values is crucial for maintaining the quality and integrity of your dataset after merging or joining DataFrames. It is important to choose a method that best fits your data and analysis goals, and to document your decisions regarding missing value handling for transparency in your analysis.
Question: Read the documentations of the Pandas DataFrame methods merge()
and concat()
, and identify the differences. Mention examples when you can use (i) either, (ii) only concat()
, (iii) only merge()
Solution:
If we need to merge datasets using row indices, we can use either function.
If we need to stack datasets one on top of the other, we can only use
concat()
If we need to merge datasets using overlapping columns we can only use
merge()
For a comprehensive user guide, please refer to the official documentation.
13.3 Independent Study
13.3.1 Practice exercise 1
You are given the life expectancy data of each continent as a separate *.csv file.
13.3.1.1
Visualize the change of life expectancy over time for different continents.
13.3.1.2
Appending all the data files, i.e., stacking them on top of each other to form a combined datasets called “data_all_continents”
13.3.2 Practice exercise 2
13.3.2.1 Preparing GDP per capita data
Read the GDP per capita data from https://en.wikipedia.org/wiki/List_of_countries_by_GDP_(nominal)_per_capita
Drop all the Year
columns. Use the drop()
method with the columns
, level
and inplace
arguments. Print the first 2 rows of the updated DataFrame. If the first row of the DataFrame has missing values for all columns, drop that row as well.
13.3.2.2
Drop the inner level of column labels. Use the droplevel()
method. Print the first 2 rows of the updated DataFrame.
13.3.2.3
Convert the columns consisting of GDP per capita by IMF, World Bank, and the United Nations to numeric. Apply a lambda function on these columns to convert them to numeric. Print the number of missing values in each column of the updated DataFrame.
Note: Do not apply the function 3 times. Apply it once on a DataFrame consisting of these 3 columns.
13.3.2.4
Apply the lambda function below on all the column names of the dataset obtained in the previous question to clean the column names.
import re
column_name_cleaner = lambda x:re.split(r'\[|/', x)[0]
Note: You will need to edit the parameter of the function, i.e., x
in the above function to make sure it is applied on column names and not columns.
Print the first 2 rows of the updated DataFrame.
13.3.2.5
Create a new column GDP_per_capita
that copies the GDP per capita values of the United Nations
. If the GDP per capita is missing in the United Nations
column, then copy it from the World Bank
column. If the GDP per capita is missing both in the United Nations
and the World Bank
columns, then copy it from the IMF
column.
Print the number of missing values in the GDP_per_capita
column.
13.3.2.6
Drop all the columns except Country
and GDP_per_capita
. Print the first 2 rows of the updated DataFrame.
13.3.2.7
The country names contain some special characters (characters other than letters) and need to be cleaned. The following function can help clean country names:
import re
country_names_clean_gdp_data = lambda x: re.sub(r'[^\w\s]', '', x).strip()
Apply the above lambda function on the country column to clean country names. Save the cleaned dataset as gdp_per_capita_data
. Print the first 2 rows of the updated DataFrame.
13.3.2.8 Preparing population data
Read the population data from https://en.wikipedia.org/wiki/List_of_countries_by_population_(United_Nations).
- Drop all columns except
Country
,UN Continental Region[1]
, andPopulation (1 July 2023)
. - Drop the first row since it is the total population in the world
13.3.2.9
Apply the lambda function below on all the column names of the dataset obtained in the previous question to clean the column names.
import re
column_name_cleaner = lambda x:re.split(r'\[|/|\(| ', x.name)[0]
Note: You will need to edit the parameter of the function, i.e., x
in the above function to make sure it is applied on column names and not columns.
Print the first 2 rows of the updated DataFrame.
13.3.2.10
The country names contain some special characters (characters other than letters) and need to be cleaned. The following function can help clean country names:
import re
country_names_clean_population_data = lambda x: re.sub("[\(\[].*?[\)\]]", "", x).strip()
Apply the above lambda function on the country column to clean country names. Save the cleaned dataset as population_data
.
13.3.2.11 Merging GDP per capita and population datasets
Merge gdp_per_capita_data
with population_data
to get the population and GDP per capita of countries in a single dataset. Print the first two rows of the merged DataFrame.
Assume that:
We want to keep the GDP per capita of all countries in the merged dataset, even if their population in unavailable in the population dataset. For countries whose population in unavailable, their
Population
column will showNA
.We want to discard an observation of a country if its GDP per capita is unavailable.
13.3.2.12
For how many countries in gdp_per_capita_data
does the population seem to be unavailable in population_data
? Note that you don’t need to clean country names any further than cleaned by the functions provided.
Print the observations of gdp_per_capita_data
with missing Population
.
13.3.3 Merging datasets with similar values in the key column
We suspect that population of more countries may be available in population_data
. However, due to unclean country names, the observations could not merge. For example, the country Guinea Bissau is mentioned as GuineaBissau in gdp_per_capita_data
and Guinea-Bissau in population_data
. To resolve this issue, we’ll use a different approach to merge datasts. We’ll merge the population of a country to an observation in the GDP per capita dataset, whose name in population_data
is the most ‘similar’ to the name of the country in gdp_per_capita_data
.
13.3.3.1
Proceed as follows:
For each country in
gdp_per_capita_data
, find thecountry
with the most ‘similar’ name inpopulation_data
, based on the similarity score. Use the lambda function provided below to compute the similarity score between two strings (The higher the score, the more similar are the strings. The similarity score is \(1.0\) if two strings are exactly the same).Merge the population of the most ‘similar’ country to the country in
gdp_per_capita_data
. The merged dataset must include 5 columns - the country name as it appears ingdp_per_capita_data
, the GDP per capita, the country name of the most ‘similar’ country as it appears inpopulation_data
, the population of that country, and the similarity score between the country names.After creating the merged dataset, print the rows of the dataset that have similarity scores less than 1.
Use the function below to compute the similarity score between the Country
names of the two datasets:
from difflib import SequenceMatcher
similar = lambda a,b: SequenceMatcher(None, a, b).ratio()
Note: You may use one for
loop only for this particular question. However, it would be perfect if don’t use a for
loop
Hint:
Define a function that computes the index of the observation having the most ‘similar’ country name in
population_data
for an observation ingdp_per_capita_data
. The function returns a Series consisting of the most ‘similar’ country name, its population, and its similarity score (This function can be written with only one line in its body, excluding the return statement and the definition statement. However, you may use as many lines as you wish).Apply the function on the
Country
column ofgdp_per_capita_data
. A DataFrame will be obtained.Concatenate the DataFrame obtained in (2) with
gdp_per_capita_data
with the pandasconcat()
function.
13.3.3.2
In the dataset obtained in the previous question, for all observations where similarity score is less than 0.8, replace the population with Nan
.
Print the observations of the dataset having missing values of population.